The following article is a writeup how a team ended up with responses of 20 seconds and being able to shorten it to 60 milliseconds. The main problem in their stack was the incorrect design of a DynamoDB table, which was solved by adding index keys to accelerate queries and drop usage of filters.
While it is very good to have such optimization, I’m left wondering how a 20 seconds response was accepted in the first place. DynamoDB clearly seems to be confusing for a NoSQL database, and looks like it’s mostly used as a starter DB for those ramping up with a microservices environment using AWS.
https://dev.to/epilot/how-we-improved-our-serverless-api-300x-3o27
This is a story about how we made a microservice response 3️⃣0️⃣0️⃣ times faster. Yes, you read that right: 3️⃣ 0️⃣ 0️⃣ times faster! 🤯
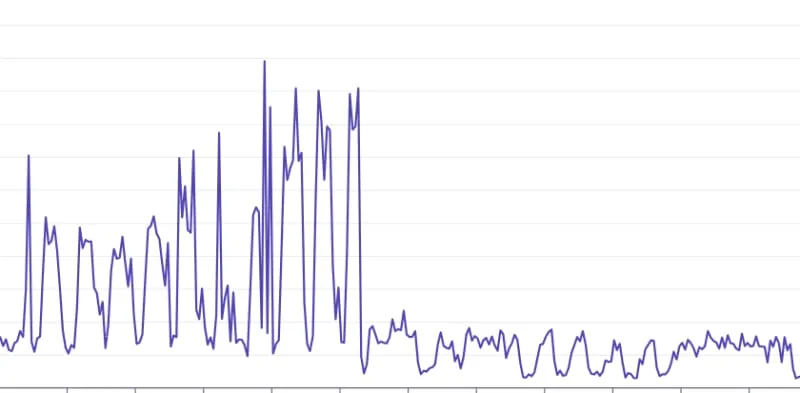
Perf improvements
We dropped the response time from an average of ~20s to ~60ms. Let me use the same scale to NOT fool your eye: 20 000 ms to 60 ms.
“Now wait a minute”, you might say. “I’m impressed you guys dropped loading times by 300X times, but what the heck 🤔 did you do to end up with such an awful response time?”
Broken promises or unrealistic expectation?
We have been heavily using AWS DynamoDB for epilot platform, mainly because of 𝟸 reasons:
- It is Serverless -> we believe in doing as little operations work as possible, when it is not the case
- It is easy to start with, when building apps with AWS Serverless technologies.
Of course we now know DynamoDB ain’t that simple as it has been promised to us. It might be attractive due to the flexibility & easy to start reasons, but designing your Microservices with DynamoDB as a storage solution, ain’t for the faint hearted 😮💨. Especially if you’re coming from an SQL heavy background, where you can flexibly query pretty much anything.
SELECT * FROM TABLE WHERE <insert clause here>
With DynamoDB, you have to design your Partition Key, Sort Key, Global & Local Secondary Indexes, in such a way, that all of your search patterns are handled optimally.
Knowing all the search patterns in advance might sound trivial at a first glance:
- find all books for an author
- find all books published in a time range
- find books by keywords,
but in a dynamic environment, such as a startup, where things can pivot from one week to another 🗓️, it can be quite a challenging endeavour, to say the least.
But now, back to the performance story.
2 important things happened which lead to such a degradation in the response times of the API:
- Designing the table without knowing the search patterns in advance.
- Increasing each record data by a 10x fold.
1.Designing the table without knowing the search patterns in advance
“Back in the day”, like the old chaps like to say, when we started building microservices, developers were aware of a good chunk of the search patterns we would have to support.
Since epilot is a multi-tenant platform, with clear separation of data between tenants, we decided to adopt DynamoDB as a storage solution, with the tenant id as the PartitionKey and the resource id as SortKey. Having this quite simplistic setup in place, we were confident we could easily query resources:
- find a resource by id:
query resources where PK=:tenantId & SK=:resourceId
- find multiple resources at once:
batchGet resources PK=:tenantId & SK=:resourceId
- query resources for a tenant:
query resources where PK=:tenantId AND {flexible_attributes_filtering}
This filter proved to bite our asses in the end. Why?
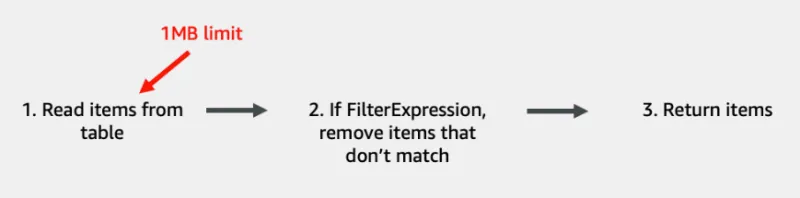
DynamoDB 1 MB limit
Well, first of all, all those {flexible_attributes_filtering} are resolved by DynamoDB after the initial query by PK=:tenantId is resolved, with a 1 MB limit. That means, DynamoDB will first match table items by PartitionKey, up to 1 MB limit, and only then apply the filter expression to further filter out returned data.
Alex Debrie - big fan of the guy btw, you should check him out, has a good article explaining, more in depth, this exact pitfall.
To quote the guy: “Filter Expressions won’t save your bad DynamoDB table design!” Oh boy, he is right! ✔️
But this didn’t prove to be lethal ☠️, until it was combined with reason number 2.
2.Increasing each record data by a 10x fold
Our resources, called workflows, were storing data about each specific started workflow (eg: name, started time, context data, status, assigned users, etc.) but also references for some data, called tasks, which was persisted & indexed by ElasticSearch, for more flexible searching.
{
"workflow": {
"name": "Wallbox",
"started_at": "2023-08-07T07:19:55.695Z",
"completed_at": "2023-08-07T07:19:55.695Z",
"status": "IN_PROGRESS",
"assignees": ["123", "456"],
"contexts": [{"id": "id1", "name": ""}, {"id": "id2", "name": ""}],
"tasks": [
{
"id": "id-1"
},
{
"id": "id-2"
},
{
"id": "id-3"
}
]
}
}
While storing those tasks in ElasticSearch, helped us support a quite flexible tasks overview dashboard in our platform, the business later decided to drop support for this feature & replace with a better dashboard 2.0.
Since ElasticSearch was no longer needed, we decided to migrate the complete data about tasks to DynamoDB, to avoid having data split in 2 storage solutions.
{
"workflow": {
"name": "Wallbox",
"started_at": "2023-08-07T07:19:55.695Z",
"completed_at": "2023-08-07T07:19:55.695Z",
"status": "IN_PROGRESS",
"assignees": ["123", "456"],
"contexts": [{"id": "id1", "name": ""}, {"id": "id2", "name": ""}],
"tasks": [
{
"id": "id-1",
"name": "Buy",
"started_at": "2023-08-05T07:19:55.695Z",
"completed_at": "2023-08-06T07:19:55.695Z",
"assignees": ["23"],
"dueDate" : "2023-09-12T07:19:55.695Z",
"status": "COMPLETED",
},
{
"id": "id-2",
"name": "Validate",
"started_at": "2023-08-05T07:19:55.695Z",
"assignees": ["73"],
"dueDate" : "2023-09-12T07:19:55.695Z",
"status": "IN_PROGRESS",
},
{
"id": "id-3",
"name": "Ship",
"started_at": "2023-08-05T07:19:55.695Z",
"assignees": [],
"dueDate" : "2023-09-12T07:19:55.695Z",
"status": "TO_DO",
}
]
}
}
Migrating all tasks data from ElasticSearch, combined with the filter expression ticking bomb, has led to times dropping significantly: from 1-3s to an average of ~20s. 💣
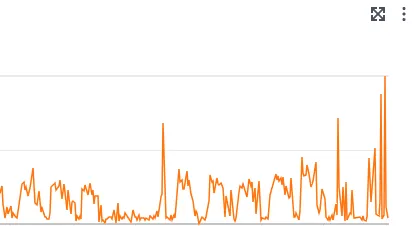
Workflows Increasing Duration
Solution
A quick investigation and the problem was spotted:
query workflows where PK=:tenantId AND contains(#contexts, :contextId)
dbClient.query({
{
// ...
FilterExpression: "contains(#contexts, :context)",
ExpressionAttributeValues: {
":context": {
"id":"id-1"
}
}
}).promise()
With the problem sitting there right in our face, it was time to implement a solution. But this time, a solution that would not turn against us in the future: better table design & good search patterns support.
In our case, that translated into storing even more data in the table. While this may sound counter-intuitive, it does help DynamoDB resolve queries much more performant.
While in the original design, only 1 table record was persisted for 1 single workflow
PK | SK | Attributes |
---|---|---|
tenantId | WF#wf1 | name, status, assignees, tasks |
tenantId | WF#wf2 | name, status, assignees, tasks |
adding extra records, for every context of the workflow,
PK | SK | Attributes |
---|---|---|
tenantId | WF#wf1 | name, status, assignees, tasks |
tenantId | CTX#ctx1 | wf |
tenantId | CTX#ctx2 | wf1 |
tenantId | CTX#ctx3 | wf1 |
tenantId | WF#wf2 | name, status, assignees, tasks |
tenantId | CTX#ctx1 | wf2 |
tenantId | CTX#ctx7 | wf2 |
helped resolve the find workflows by context query a no of 10-20 X faster ⚡️.
1. Find workflow ids by context
dbClient.query({
{
// ...
KeyConditionExpression: `PK=:tenantId AND begins_with(SK, :ctx)`,
ExpressionAttributeValues: {
':tenantId': tenantId,
':ctx': `CTX#id`
}
}).promise()
This would return the list of workflow ids by a specific context id.
const ids = [{PK: tenantId, SK: wfId1}, {PK: tenantId, SK: wfId2}, ...]
1. Batch get workflows by ids (\*)
dbClient.batchGet({
{
RequestItems: {
[TABLE_NAME]: {
Keys: [{PK: tenantId, SK: wfId1}, {PK: tenantId, SK: wfId2}, ...]
}
}
}).promise()
(*) - batch get queries must be limit to 100 records, as per AWS Documentation
Conclusions
We learned our lessons. We did it the hard way. But we did it, nonetheless. 📝
While DynamoDB sounds great for the schema-less flexibility, at the end of the day, you have to do your homework and understand all the search patterns you plan to support.
Simply relying on Filter Expressions to save your day is not gonna cut it.
A good design is key 🗝️ to the success of your application!